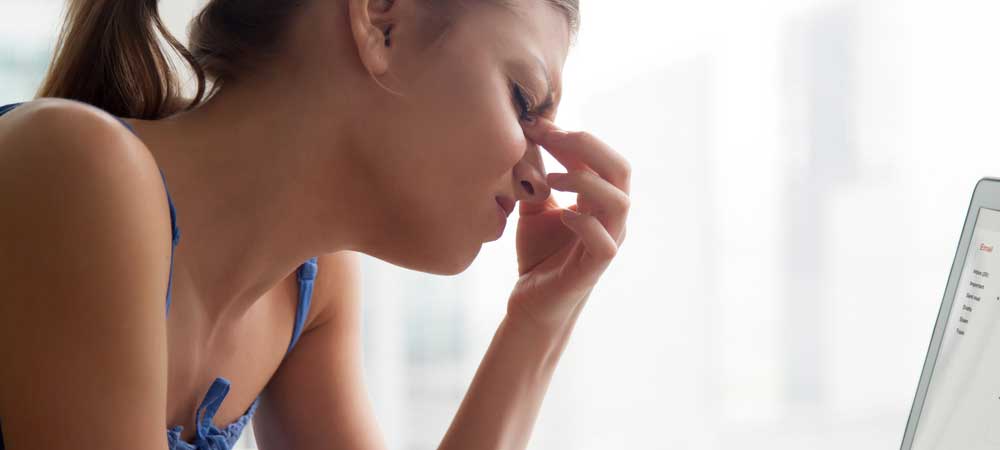
Unquestionably, the advent of artificial intelligence (AI) has signaled a transformative shift in numerous sectors. One of the most compelling areas of impact has been healthcare, with a particular focus on the advancements in visual field testing, a crucial component in diagnosing and managing ocular conditions such as glaucoma.
AI, equipped with machine learning algorithms, has an unparalleled ability to process large data sets with high precision, making it a valuable tool in the analysis of visual field tests. Traditional methods for visual field testing, such as the Humphrey Visual Field Analyzer, require patient interaction and are subject to variability and inconsistency. AI steps in to alleviate these concerns by providing objective, reliable, and comprehensive analysis.
Among the most remarkable benefits of AI in visual field testing is the potential for earlier detection of eye diseases. Machine learning algorithms can detect patterns and subtle changes that are easily overlooked by human observation, enhancing the early diagnosis of conditions like glaucoma. Early detection allows for timely intervention, which can prevent vision loss and improve the patient’s prognosis.
AI also presents opportunities for enhanced efficiency. Visual field tests typically require skilled interpretation by eye care professionals. By incorporating AI, we can reduce the time taken to interpret results, freeing up healthcare professionals to focus more on patient care. Additionally, machine learning models can learn from previous visual field data, helping optimize testing procedures and ultimately reducing the time patients spend undergoing these tests.
Notably, AI can facilitate more personalized treatment plans. By processing and understanding individual patterns in visual field changes, AI can predict disease progression at the individual level, allowing for tailored treatment strategies.
Despite these exciting prospects, the application of AI in visual field testing does not come without limitations. A significant concern is the reliance on good-quality data. The accuracy of AI models is heavily dependent on the quality and diversity of the data input. Thus, if the data is biased or inadequate, the AI could produce inaccurate predictions.
Furthermore, there is concern about the interpretability and transparency of AI. As these algorithms become more complex, it becomes more challenging to understand their decision-making process. This ‘black box’ problem can pose issues when trying to validate and trust AI’s decisions.
Additionally, integrating AI into current healthcare systems presents logistical challenges, requiring considerable resources, including training for healthcare professionals to properly understand and use AI.
Moreover, ethical and legal considerations surround AI. For instance, who is responsible if an AI system makes an incorrect prediction that leads to patient harm?
AI is changing the landscape of visual field testing, offering several benefits that promise to enhance early detection, efficiency, and personalization of care. However, the journey is not without hurdles. Ensuring high-quality data, addressing the ‘black box’ problem, and dealing with ethical, legal, and logistical issues are crucial challenges to overcome. As we navigate through these, we must strive to ensure that the potential of AI in visual field testing is harnessed responsibly and effectively. The future is indeed in sight, and with careful steps, it looks promisingly bright.